Climate datasets
While there is a variety of rainfall datasets for Eritrea, few are suitable for analyses of long-term trends and variability. In this exercise, we will use the CMAP rainfall estimates and NDVI version g presented in the Find Data Sources section.
In order to compare the malaria incidence data versus the climate/environmental factors, the seasonality should first be removed by computing monthly anomalies (departure from long term mean).
Anomalies are used instead of recorded values because both climate variables and malaria incidences have a similar seasonal pattern. Statistical analysis of recorded values would indicate a very high correlation between climate and malaria incidence. This is only due to the fact that the temporal evolution of climate variables and malaria incidences follow the same seasonality. In order to eliminate the seasonal cycle effect and study only the impact of variations in climate with variations in malaria incidence, the long-term mean is subtracted from the recorded data.
An anomaly is computed as the difference between the value of either rainfall or vegetation and its climatological mean value. Monthly anomaly is computed as the difference between the original monthly value in a given month and the monthly climatological value for that month of the year.
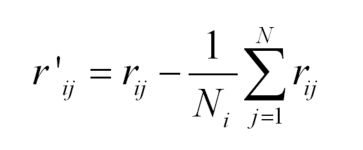
Here, for months i and years j , r’ij is the monthly anomaly and rij is the original monthly value. The remainder of the equation is the calculation of the monthly climatology, which is subtracted from the original monthly values.
The monthly anomalies, both for climate environmental variables and for incidences data, are used for the analysis.
The satellite-derived climate variables from the IRI data library must be averaged over the same administrative boundaries as the incidence data, using subzoba boundaries. Whilst it would have been preferable to use smaller boundaries such as health facility catchment areas, these are not yet available.